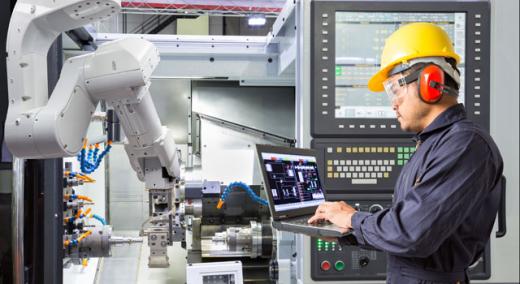
Body
‘Process Capability: What It Is and How It Helps,” parts one, two, three, and four, discussed Alan’s development in the field of process capability1 He’d learned about the mistakes that can be made and how to avoid them in practice to become better at his job. Alan had since passed on his learning to colleagues, one of whom, Owen, had led some successful assessments of process capability.
…
Want to continue?
Log in or create a FREE account.
By logging in you agree to receive communication from Quality Digest.
Privacy Policy.
Comments
Use of Capability Indices
Scott, your articles on the use of control charts and the importance of process stability are superb, as well as foundational to proper quality management.
However, the use of capability indices, Cp, Cpk, Cpm, etc. are remnants of a desire to meet requirements, likely in pursuit of a goal of zero defects, thinking which continues to dominate most quality management practices. Lost in these efforts is the difference between a process which is in control and on target, as proposed by Dr. Genichi Taguchi, and having a Cpk of 2 (Six Sigma Quality) and being off target. Also lost is what it means to continuously improve, past zero defects, or exceeding a Cpk of 2.0. Then again, the economics of using Dr. Taguchi's Quality Loss Function may suggest that continued improvement is not economically justified.
For additional reference, here's a closing statement from "The New Economics," Dr. Deming's last book,
"This simple illustration should put to rest forever use of measures of dispersion like Cpk, as it has no meaning in terms of loss. Moreover, it can be decreased [actually, a typo, should be "increased"] to any value merely by widening the specifications. Conformance to specifications, Zero Detects, Six Sigma Quality, and other (specification-based) nostrums, all miss the point (so stated by Donald J. Wheeler. 1992.
In closing, Dr. Deming proposed that quality management could be dramatically improved by moving away from decision-making on the basis of process capability indices of any kind and towards awareness of the economics offered by thinking in terms of a Taguchi Loss Function. And, for those use "target thinking" in their wood working projects at home, as when they scribe a line and cut the wood to be as close as possible to this single line, let this be a reminder that they are not using tolerances to cut "anywhere in between." Could it be that they well know that the closer the length of wood is cut to the scribed line, the easier will be their assembly operations? Why not offer this advantage to co-workers as well and shift quality management from the management of defect-free parts to the management of variation in the system of parts or any product or service, guided by the use of control charts?
Add new comment