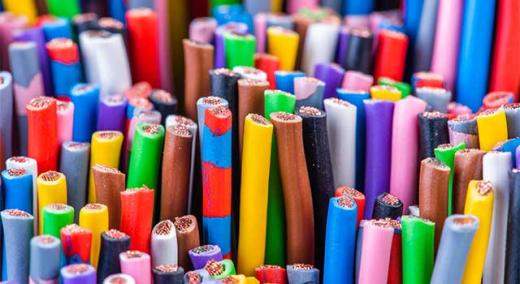
The cumulative sum (or Cusum) technique is occasionally offered as an alternative to process behavior charts, even though they have completely different objectives. Process behavior charts characterize whether a process has been operated predictably. Cusums assume that the process is already being operated predictably and look for deviations from the target value. Thus, by replacing process characterization with parameter estimation, Cusums beg the very question process behavior charts were created to address.
ADVERTISEMENT |
To illustrate the Cusum approach and compare it with an average chart, I’ll use the example from page 20 of Shewhart’s first book, Economic Control of Quality of Manufactured Product (Martino Fine books, 2015 reprint).These data consist of 204 measurements of electrical resistivity for an insulator. Shewhart organized them into 51 subgroups of size four, based upon the time order in which the measurements were obtained. Figure 1 gives the averages and ranges for these 51 subgroups.
…
Comments
Wonderful articles
These are just wonderful articles. I used Dr. Wheeler's simple, easy to understand books for some 35 years to bring order out of chaos. Thanks.
The Simplest Solution is Often the Best Solution
It's hard enough to get people to use and understand simple X and R charts. Complicating the calculations with other charts makes prospective users run for the hills.
Dr. Wheeler continues to demystify SPC and point us in useful directions. Thanks!
Process Behavior Chart vs Control Chart
I never miss reading a Dr. Wheeler article in Quality Digest. They are always insightful and challenging.
I understood that Dr. Wheeler uses the term process behavior chart instead of Dr. Shewhart's term control chart. Am I incorrect?
If I am correct, I am thrown off by the use of the material target value of 4,000 for the center line of average chart rather than the grand mean of 4,498. Page 20 of Economic Control of Quality of Manufactured Product used 4,498.
I would appreciate any insight into my misunderstanding.
Thanks
Reply for Jonathan Boyer
Normally we would use the average of 4498 as the central line. However, since Cusum is a technique for detecting deviations from a TARGET value, I used the target value of 4,400 on the Average chart to make the comparison between the two twchniques more equitable
Process Behavior Chart vs Control Chart
Dr. Wheeler, Thanks for clearing that up for me. Your article did say that, but I did not grasp it when I read the article.
Thanks again,
Jonathan
Thank you for the article in understandable human language.
Thank you for the article, dear Danald
Add new comment