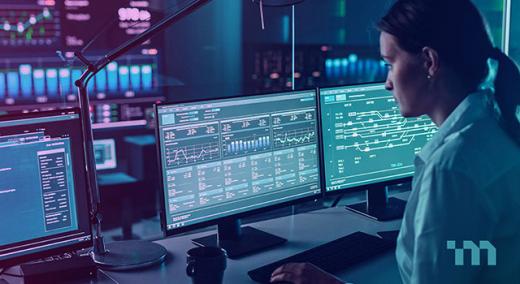
In April 2018, the U.S. Food and Drug Administration (FDA) permitted the marketing of the first medical device to use artificial intelligence (AI). The device, called IDx-DR, is a software program that uses an AI algorithm to analyze images of the eye taken with a retinal camera called the Topcon NW400. A medical professional uploads the digital images of the patient’s retinas to a cloud server where the IDx-DR software is installed. The software then provides the doctor with one of two results: Refer the patient to an eye care specialist, or rescreen in 12 months. Overall, the technology accelerates diagnosis and treatment of diabetes-related damage to the retina. This is one example of how medical devices using AI are traversing regulatory pathways into mainstream healthcare.1
ADVERTISEMENT |
The concept of AI has been around and progressing in linear fashion for decades. In one scenario, the technology was referred to as artificial neurons capable of performing logical functions. During the early 1950s, computer scientists developed AI programs that could best their human counterparts at games of chess and checkers. While this might be a highlight at parties, the innovation of this technology went far beyond board games.2
…
Comments
AI Data Handling and Ethical Framework for AI
Hi Mr Talpade, thanks for mentioning our IDx-DR product! Our (very) recent paper describing an ethical framework for AI with regulatory considerations - 2nd, 3rd and 4th authors are all FDA Directors - may be of interest to your readers:
https://www.aaojournal.org/article/S0161-6420(21)00643-6/fulltext
Add new comment