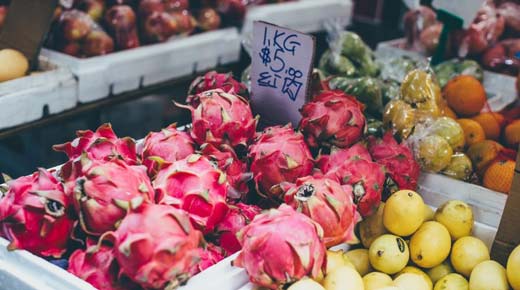
The simplest type of process behavior chart is the chart for individual values and a moving range. It allows us to plot a point every time we get a value, making it perfect for data that occur one value at a time. A brief history of this simple chart follows.
In the 1920s, Walter A. Shewhart developed “control charts” as a technique for analyzing observational data. Today we use the more descriptive name of process behavior charts. The original process behavior chart for measurements used a series of subgroups containing two or more original data. The original chart plotted the averages and root mean square deviations of each subgroup. In practice this quickly became the average and range chart we know today. The fundamental concept that makes this chart work is the notion of rational subgrouping. According to Shewhart, when we place two values together in the same subgroup we are making a “judgment” that these two values were collected under “essentially the same conditions.” Thus, the objective of rational subgrouping is to end up with homogeneous subgroups.
…
Add new comment