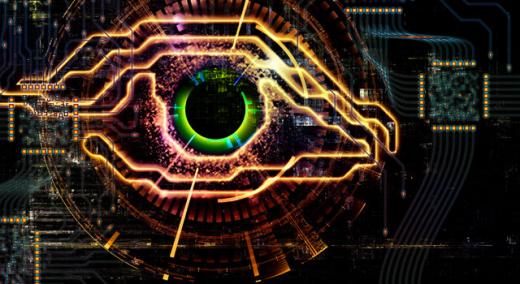
This article is an extract from Smart Manufacturing, the Lean Six Sigma Way, Wiley, available May 2022
The U.S. and EU economies are facing a major labor shortage in manufacturing. A pre-Covid survey projected the need for more than four million new manufacturing jobs by 2030, but with a shortfall of more than two million. Given the growing labor shortage caused by Covid-19, the problem can be expected to worsen.2 The shortage in quality assurance will be even more critical as these positions require dedication and advanced skills beyond what many factory workers have. Even with the most dedicated and skilled inspectors, unacceptable error rates are common occurrences as people get distracted and fatigued.
…
Comments
Visual Inspection Effectiveness
Dear Sir, I would encourage you to read further on human visual inspection effectiveness by obtaining the text, "Improving the Effectiveness of Visual Inspection" published by American Foundry Society in 2018. I would further suggest that your thoughts be refined by the several peer-reviewed journal articles on the subject I have written for AFS Transactions, available in the on-line library of American Foundry Society (www.afsinc.org). A recent paper, "Quality Management Perspectives on the Use of Machine Vision and AI-Guided Inspections," published in 2021, would be of direct relevance to your column.
Add new comment