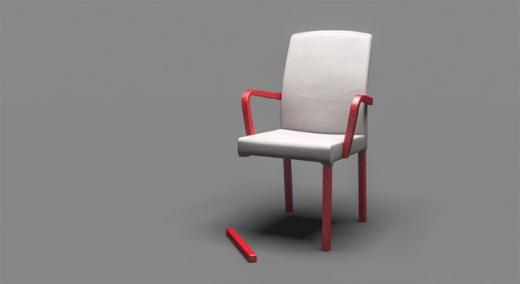
Body
In a general sense, capability is the ability to do something. Within manufacturing, capability is given a much more specific definition. It is an expression of the accuracy of a process or equipment, in proportion to the required accuracy.
ADVERTISEMENT |
This can be applied to production processes, in which case any random variation and bias in the process must be significantly smaller than the product tolerance. It can also be applied to measurements, where any uncertainties in the measurement must be significantly smaller than the product tolerance or process variation that is being measured.
…
Want to continue?
Log in or create a FREE account.
By logging in you agree to receive communication from Quality Digest.
Privacy Policy.
Comments
Process capability
I must take exception on your first two sentences.
Capability means what it means, “the extent of someone's or something's ability.” Please do not try to add QA concepts to the word when we already have a phrase to cover it, “Process Capability.” Even process capability is too restricting for what you are attempting to explain. “Capability Ratio”, and “Capability Index” both are specific to the concepts you use.
In today’s manufacturing world no one can take a risk and attempt to satisfy a customer’s new order is the equipment will not be capable to produce it. Note, I said “will not,” future tense.
Businesses need to know now, what their processes are capable of to a capability index that is at least acceptable to industry standards. It is far too late to be awarded the contract and find the Cpk is only 0.43.
The better way to start is to keep track of all the feature variances of all the processed orders run on the machine. These can be pooled into like groups, very similar to group technology coding, to define the pooled variance of this feature, in this material, using these controls. Using this pooled variance, a standard deviation can be calculated. This multiplied by 6 + (1.33 x 2) provides a true capability for that process in particular.
How, you ask? By working the Cpk calculation backwards. All processes may drift 1.33 standard deviations from the mean in regular process mode. This represents the Cpk of 1.33 that most businesses’ expect. It also means higher AND lower, so we have to allow for it twice. Hence 1.33 x 2. Most industries expect 6Σ (± 3 µ) performance from their vendors and suppliers, so we have 6 + (1.33 x 2) or 8.66.
This not gives a full capability range to judge new work against before quoting. If the work is in Aerospace change the multiplier from 8.66 to 14.66 for ±12Σ. If the industry isn’t that critical, maybe go with 6.66. Do what makes sense for YOUR industry and customer expectations.
If your capability is not robust enough to satisfy either your industry or (more importantly) your customers, undertake a CI program to find the critical points that improve the outcome and control them.
World class manufacturers know these things ahead of time and make their managerial and business decisions based on them. That is why they have world class profits too.
Add new comment