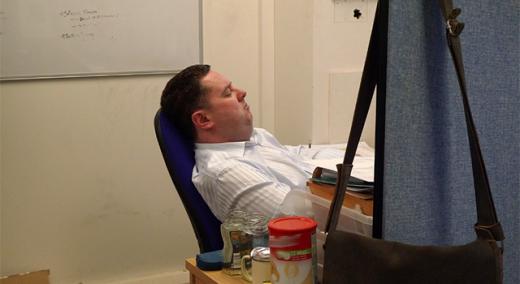
Image: Henry Burrows
‘What’s the MTBF of a human?” A bit of a strange question I ask in my Reliability 101 course. Why ask such a weird question? I’ll tell you why. Because MTBF is the worst, most confusing, crappy metric used in the reliability discipline.
ADVERTISEMENT |
OK, maybe that statement is a smidge harsh, but it does have good intentions because the amount of damage done by misunderstanding MTBF is horrendous.
MTBF stands for “mean time between failure.” It is the inverse of failure rate. An MTBF of 100,000 hours/failure is a failure rate of 1/100,000 fails/hour = .00001 fails/hour. Those are numbers; what does that look like in operation?
Does it mean:
The product lasts 100,000 hours before failing?
Half the population fails by 100,000 hours?
Wait a minute! Our product is only supposed to last three years with a 50-percent duty cycle. That’s 13,140 hours of use. Why would we have an MTBF goal of 100,000 hours? It can’t even run that long if everything goes perfectly.
…
Comments
Very useful and clear,
Very useful and clear, thanks!
"No MTBF" blog link
Hi ... I am teaching a Reliability course in the fall. Thanks for the article; I will be able to use the content in class. I was interested in reading more about this subject on the "No MTBF" blog, but the link doesn't seem to work. Could you repost the address to this blog? Thanks! Diane
Linnk
Hi Diane
The link is http://nomtbf.com/2020/03/mtbf-of-a-human/
Add new comment