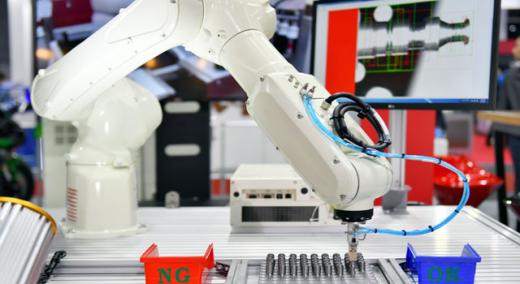
The automotive industry is a critical part of the global economy, and the quality of the products it produces is essential for its success. Consumers demand high-quality vehicles that meet their needs, and companies in this industry must ensure that their products meet rigorous safety and performance standards. Quality control is therefore essential for automotive manufacturers, and automakers are constantly looking for faster, more accurate, and more human-free methods for inspection.
ADVERTISEMENT |
As it has in other industry applications, artificial intelligence (AI) is making inroads into automotive assembly and inspection. However, a major stumbling block is creating the training data required for AI inspection models. Defects are rare, and thus it’s hard to collect relevant imagery to train AI. It’s also expensive and time-consuming to annotate images. The automotive industry needs a better solution for collecting training data.
…
Add new comment