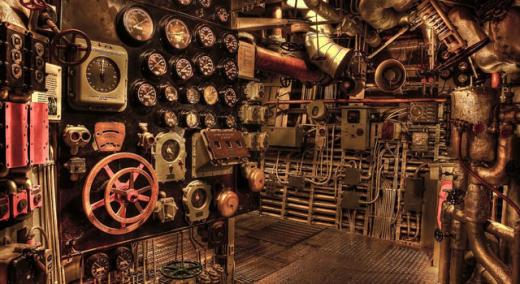
When we talk about measurement system analysis (MSA), people tend to focus on attribute agreement analysis because it is usually quicker and easier to do than a gauge repeatability and reproducibility (gauge R&R) study. This article is a review of the fundamentals for gauge R&R to remind us why it is so critical. We will review the basic definitions, go through a process for preparing a study, and then review the output in Minitab to make sure we understand what is going on in the analysis.
ADVERTISEMENT |
Why do we do a gauge R&R study in the first place? We do it for two reasons. One is to validate that the measurement process is acceptable. The second is to feel comfortable about the data. We want to avoid the potential for embarrassment by presenting data in a meeting and having it challenged. We want to be able to show what we did to validate it and to have confidence the data are good. Doing MSA also helps us to understand what is in the collection process and helps convince the people collecting the data why it’s important to do so. If the data collector understands why the study is being done, then he will be more likely to identify problems when they occur.
…
Comments
Comments on your study examples
Hi,
Thanks for the article, a few observations on the examples.
This may be a terminology aspect but I think its very important, you mention in your wording for example 1 that the issue relates to the consistency of the measurement process, yet the range chart indicates that the measurement process is consistent, if nothing would change it could go on measuring at this level.
The fact that the measurement system, based upon this study cannot distinguish between your chosen parts is evident from the X Bar Chart, in fact there's no point in looking at any other numbers if this is important because all the part values are plotted within the control limits which contain measurement variation, in other words we are measuring in the noise.
In example 2 you change the method to standardise the part preparation which gives the measurement process more of a chance to not only distinguish between parts (you may of changed your part selection too) but has resulted in decreasing the measurement variation as depicted by the lowered standard deviation.
By looking at the X Bar chart associated with example 2 now we can see that the observations are all outside the control limits therefore based on this selection of parts we can be comfortable that we can distinguish between them. Note that the R Chart still shows operator consistency, we may not be comfortable with the measurement error but we can be confident that it can be replicated.
If we need to further improve we can always look at systematic detectible differences between the operators by using other methods.
Out of interest, what are the number of replicates per part here in order for the software to calculate sd's of 2.31 and 0.889 for the two examples?
Thanks
Add new comment