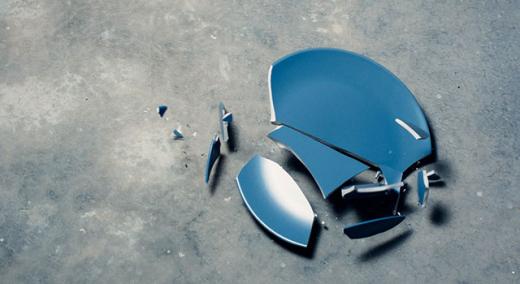
A hypothesis-driven approach to problem solving and making recommendations can be tremendously efficient. You create a hypothesis (i.e., something taken to be true for the sake of argument), conduct analysis designed to prove or disprove the hypothesis, then make your recommendation based on the results of your analysis. Typically, your hypothesis is based upon prior experiences you’ve had as well as your knowledge of the subject matter you’re evaluating.
ADVERTISEMENT |
I’ve personally used this approach for years. I refer to it as the structured thought process. The method is both efficient and effective. That said, using this approach is not without risks.
Risk No. 1: Confirmation bias
Although it’s great to have experience and prove your hypotheses are correct, that same experience carries risk with it. Confirmation bias—the tendency to look for or interpret information in a way that confirms your preconceived ideas—is the biggest risk you face when using a hypothesis-driven approach like the structured thought process.
…
Comments
Your parenthetical phrase
Dude that's an assumption, not a hypothesis.
Merriam-Webster definition
Definition of "hypothesis" from Merriam-Webster
1a: an assumption or concession made for the sake of argument
b: an interpretation of a practical situation or condition taken as the ground for action
2: a tentative assumption made in order to draw out and test its logical or empirical consequences
Analysis mistakes
It seems that there is more to this subject than what is presented. More data is not analysis paralysis. Traditionally hypothesis testing is to take a sufficient sample size to make a decision from the test to minimize false positive or false negative conclusions about the population. This article focuses on "accepting" an hypothesis. That means having sufficient data to avoid a false positive conclusion of accepting a result incorrectly. It also depends on the statistical tools for Big Data that one uses to handle large data sets, not analysis paralysis. (see Duarte, J, "Data Disruption", Quality Progress, Vol. 50, Issue 9, Sept. 2017) There are other topics in this article worth commenting on, but I chose to address only one at this time.
RISK ANALYSIS
I AGREE WITH YOU
THE ANALYSIS IN POSITIVE DIRECTION IS IMPORTANT
INTERPRITATION OF ANALYSIS AND CORRECTIVE ACTION IS VERY IMPORTANT . RELATION BETWEEN PRODUCTION , QC AND ANALYSIST IS IMPORTANT
anup.chandra@yahoo.com
Add new comment