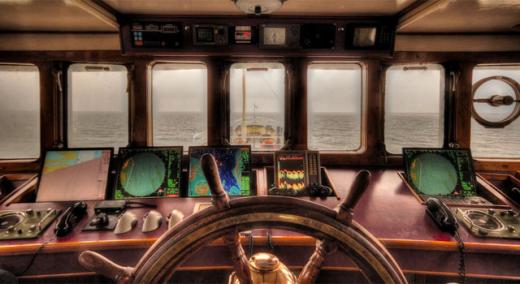
After reviewing Mark Graban’s wonderful book, Measures of Success (Constancy, 2018), I started rereading Walter Shewhart’s books, Statistical Method From the Viewpoint of Quality Control (Dover reprint 1986, originally edited by W. Edwards. Deming), and Economic Control of Quality of Manufactured Product (Martino Fine Books, 2015 reprint). Both are excellent books for any quality professional.
ADVERTISEMENT |
One of the themes that stood out for me while reading the two books was the concept of cybernetics. This column is a result of studying Shewhart’s books as well as articles on cybernetics by Paul Pangaro.
…
Add new comment