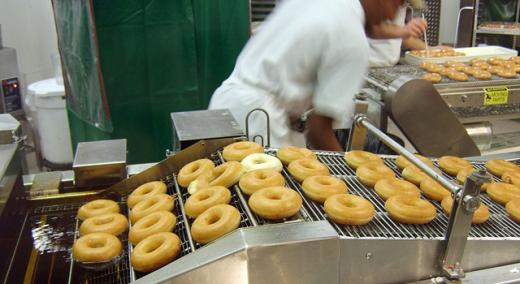
It has been a while since I have written about statistics, and I get asked a lot about a way to calculate sample sizes based on reliability and confidence levels. So today I am sharing a spreadsheet that generates an operating characteristic (OC) curve based on your sample size and the number of rejects. The spreadsheet (there's a link to it at the end of this article) should be straightforward to use. Just enter your own data in the required yellow cells.
ADVERTISEMENT |
A good rule of thumb is to use a 95-percent confidence level, which also corresponds to 0.05 alpha. The spreadsheet will plot two curves. One is the standard OC curve, and the other is an inverse OC curve. The inverse OC curve has the probability of rejection on the y axis, and the percent conforming on the x axis. These correspond to confidence level and reliability, respectively.
I will discuss the OC curve and how we can get a statement that corresponds to a reliability/confidence level from the OC curve.
…
Comments
Great Discussion on Reliability and Confidence
Thanks for the brief but powerful article on reliability and confidence in sampling. Always timely. And also for the spreadsheet / calculator.
Add new comment