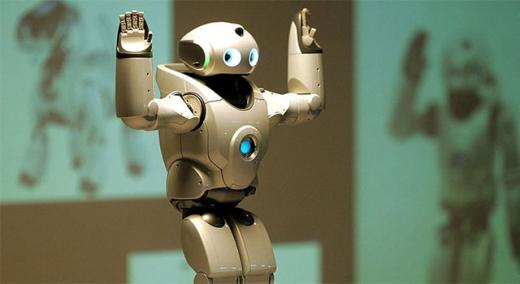
Although we’re still a few decades out from seeing most businesses and industries use artificial intelligence (AI) in their operations, there is certainly greater adoption than there has been in years past. It behooves business leaders to learn all they can about AI.
ADVERTISEMENT |
According to a top IT training company, 2021 saw a sharp uptick in respondents to its annual AI Adoption in the Enterprise survey, (nearly three times than in the previous year), as well as 61 percent of respondents showing that they are actively considering or evaluating AI solutions.
If you are interested in learning more about AI, here are four things you should know.
AI is already embedded in people’s day-to-day
While widespread and more sophisticated uses of artificial intelligence are perhaps slated in the not-so-distant future, people are already using or interacting with some form of AI every day. If you have used web-based email services, used ridesharing services, or shopped on e-commerce platforms, you’ve likely been interacting with artificial intelligence in some capacity and didn’t know it.
…
Comments
Well presented....
A quotable quote:
"AI solutions cannot fix antiquated or poor internal processes and systems. Throwing AI solutions on top of flawed systems will not only lead to failure but also quicker failure as well as huge tangible and intangible costs."
It is that decades old maxim: "Garbage in, garbage out..."
Add new comment