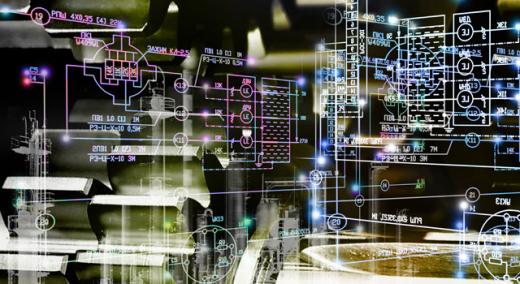
There are two ways to increase profits: increase sales or reduce costs. Although most data analysis seeks to find more ways to sell more stuff to more people, addressing preventable problems is an often overlooked opportunity. Preventable problems consume a third or more of corporate expenses and profits.
ADVERTISEMENT |
Data analysis can pinpoint problems and eliminate them forever. Problem solving with data is a much more reliable and controllable way to cut costs and increase profits. Sadly, few people know how to do this consistently.
How do you solve operational problems with 100-percent success rate? Take out the guesswork. The vast majority of improvement projects involve reducing or eliminating defects, mistakes, and errors. If you have raw data about when the defect occurred, where it happened, and what type of defect it was, you can create a world-class improvement project that eliminates the guesswork. And you can do it using a tool you most likely already have: Microsoft Excel.
…
Comments
Excel Pivot Tables and Data Analysis
Jay:
Excellent article- very helpful.
I was trained in data analysis in the mid-eighties. We used the approach you outline-but the number crunching was tedious with the earlier software. The evolution of Excel spreadsheets improved the process. The application of pivot tables you describe looks to be quite efficient.
It is interesting that you described an important concept (DMAIC: Define, Measure, Analyze, Improve, Control) of Six Sigma, without referencing Six Sigma. The approach you outlined pre-dated Six Sigma, but is frankly just an organized application of common sense problem solving using data.
I have been disappointed recently, observing how 'quailty professionals' have been vilifying Six Sigma. While there have been some misapplications- and overstating the successes of Six Sigma, the tools such as DMAIC can be very helpful in problem solving and continual improvement as you describe in your article.
Nice job!
Milt
Add new comment