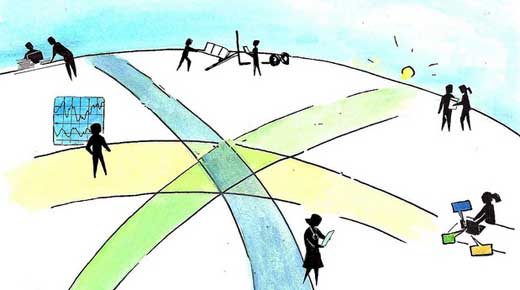
The data you've already collected can be reused for further process improvements
Credit: IBM Curiosity Shop
Plant-floor quality issues tend to focus on a company’s technical resources. When products fall out of spec, alarms sound and all hands are immediately on deck to fix things. Despite large technology investments to monitor and adjust production processes, manufacturers are still bedeviled by quality problems. The issue is not a lack of technology. It is a lack of quality intelligence.
ADVERTISEMENT |
When problems occur, manufacturers must obviously fix them. But the typical organization expends much more energy reacting to problems rather than preventing them. This is true despite our understanding that, “an ounce of prevention is worth a pound of cure.” We know that proactive measures can be immensely profitable, and yet our limited quality resources spend little time identifying strategic imperatives for avoiding problems. Instead, most of their time is spent responding to issues. Today’s quality professionals are too preoccupied with just fighting the fires that rage on shop floors.
…
Add new comment