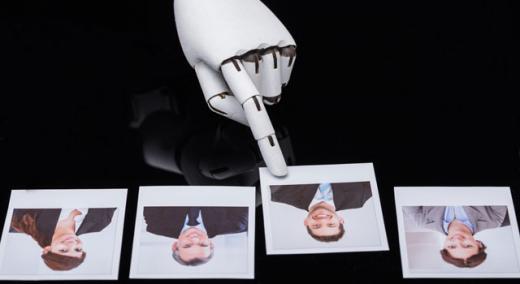
Body
With ongoing global shortages of all kinds of goods, from cars to lumber to cooking oil, both consumers and companies are struggling. The Russian invasion of Ukraine, along with renewed Covid outbreaks in China, are two things that are likely to make things worse before they get better.
…
Want to continue?
Log in or create a FREE account.
By logging in you agree to receive communication from Quality Digest.
Privacy Policy.
Add new comment