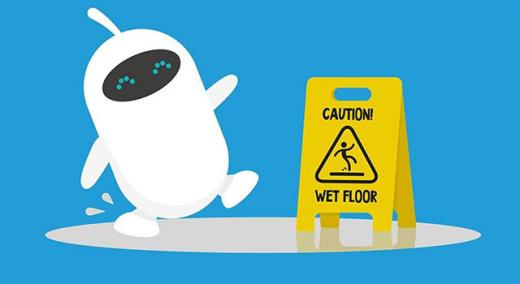
Do you see the limitations and over-hyped expectations of today’s approach to artificial intelligence (AI)? Does it need a reboot, a redirection, to finally achieve its potential, one that truly understands us and we can trust?
ADVERTISEMENT |
That is the premise of a great book on the subject, Rebooting AI: Building artificial intelligence we can trust (Vintage, 2019) from Gary Marcus and Ernest Davis. Marcus is both a professor of psychology at New York University and co-founder of robust.ai. Davis is a professor of math at New York University and one of the world’s leading scientists on commonsense reasoning for AI.
A quote on the book’s cover by chess master Garry Kasparov nicely sums up why this book is needed: “Finally, a book that tells us what AI is, what AI is not, and what AI could become if only we are ambitious and creative enough.”
…
Add new comment