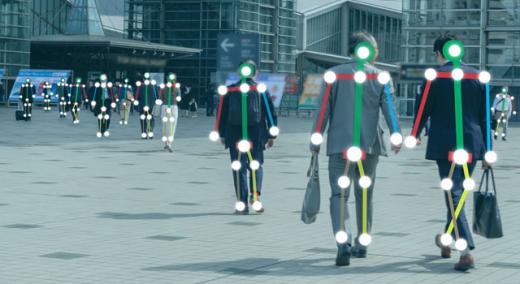
One of the biggest problems when reading about any kind of innovation in the press is the prevalent assumption that everyone understands the topic and how it works. Whether it’s cloud computing, edge computing, cold fusion, controlled fusion, or recently, artificial intelligence (AI), experts and reporters start extolling the technological miracle without offering even a simple explanation about what it is.
ADVERTISEMENT |
Articles about AI have been especially annoying, in part because of its name—AI is neither artificial nor intelligent—but also because it conjures up images of machines that think and reason, which is far from the reality.
When Jeff Dewar, CEO of Quality Digest, was at MasterControl’s Masters Summit 2022 in Salt Lake City, he had a chance to sit down with Viktoria Rojkova, MasterControl’s vice president of AI/ML and Data Science, and ask her to explain just what AI is. Her answers were clear, concise, and easy to understand.
Although the ultimate goal of AI is to create machines that perform tasks that would normally require human intelligence, first we must teach machines to learn. In other words, machine learning (ML) is used to achieve AI.
…
Comments
Narrow AI for Quality Improvement Projects
Narrow AI limits itself to a single task.
Years ago, I found ways to use Excel PivotTables to digest large amounts of defect data and turn it into improvement projects consisting of control charts, Pareto charts and Ishikawa diagrams.
Doing it manually took many hours to many days, but that analysis was the source of many multi-million dollar improvement projects.
This is where the invisible low-hanging fruit exists in any company.
I later automated the analysis to collapse the time into just a few minutes.
Taking a repetitive human task like data analysis and automating it isn't full-blown AI, but it's a step in the right direction.
Add new comment