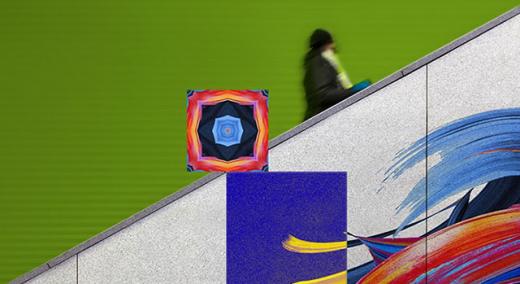
With big data and artificial intelligence (AI) transforming business, it’s almost certain that every executive will need to leverage these technologies at some point to advance their organization—and their career. However, doing so carries a heavy intimidation factor for most leaders, and this is often exacerbated by skill-heavy job descriptions for leadership roles related to data, analytics, or AI. However many of these descriptions misunderstand what’s required to drive successful business outcomes using data and AI.
ADVERTISEMENT |
Although analytical thinking is certainly important, many traditional leadership skills are equally essential when undertaking AI/big data for the first time. It’s also critical to be comfortable with ambiguity, have the capacity to drive consensus among disparate players, and understand the levers of value to prioritize accordingly. Some of the most effective leaders in data and AI are those who think commercially while applying expertise. Moreover, today’s data-driven business leaders must be politicians and communicators, able to harness the potential of data and AI to drive revenue, efficiency gains, and innovation, while exerting influence and explaining the value they create.
…
Add new comment