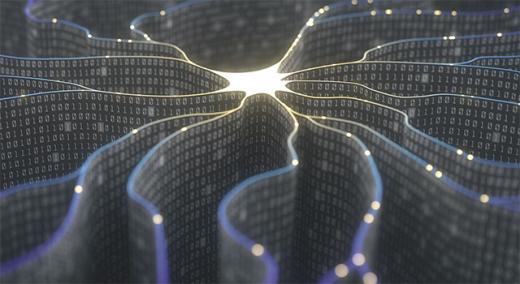
Oak Ridge National Laboratory researchers used an invertible neural network, a type of artificial intelligence that mimics the human brain, to select the most suitable materials for desired properties, such as flexibility or heat resistance, with high chemical accuracy.
Astudy led by researchers at the U.S. Dept. of Energy’s Oak Ridge National Laboratory could help make materials design as easy as point-and-click.
ADVERTISEMENT |
The study, published in Nature Partner Journals’ Computational Materials, used an invertible neural network, a type of artificial intelligence that mimics the human brain, to select the most suitable materials for desired properties, such as flexibility or heat resistance, with high chemical accuracy. The team’s findings offer a potential blueprint for customizing scientific design and speeding up the journey from drawing board to production line.
…
Add new comment