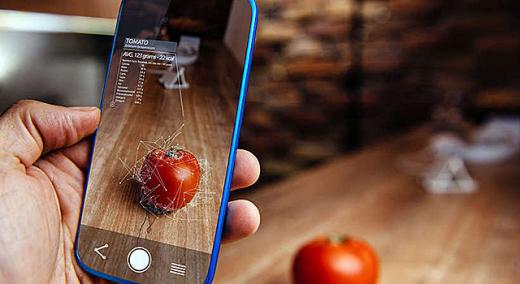
Adobe Stock
As artificial intelligence (AI) becomes increasingly ubiquitous in various industry sectors, establishing a common terminology for AI and examining its various applications is more important than ever. In the international standardization arena, much work is being undertaken by ISO/IEC’s joint technical committee JTC 1—Information technology—Subcommittee SC 42—Artificial intelligence, to establish a precise and workable definition of AI. Through its working group WG 4, SC 42 is looking at various use cases and applications. The convener of SC 42/WG 4 is Fumihiro Maruyama, senior expert on AI at Fujitsu Laboratories.
ADVERTISEMENT |
Currently, there are a total of 70 use cases that the working group is examining. Health, for example, is a fascinating area to explore. Maruyama himself describes one use case in which a program undertakes a “knowledge graph” of 10 billion pieces of information from existing research papers and databases in the medical field. The application then attempts to form a path representing the likely development from a given gene mutation to the disease that deep learning has predicted from the mutation.
…
Add new comment