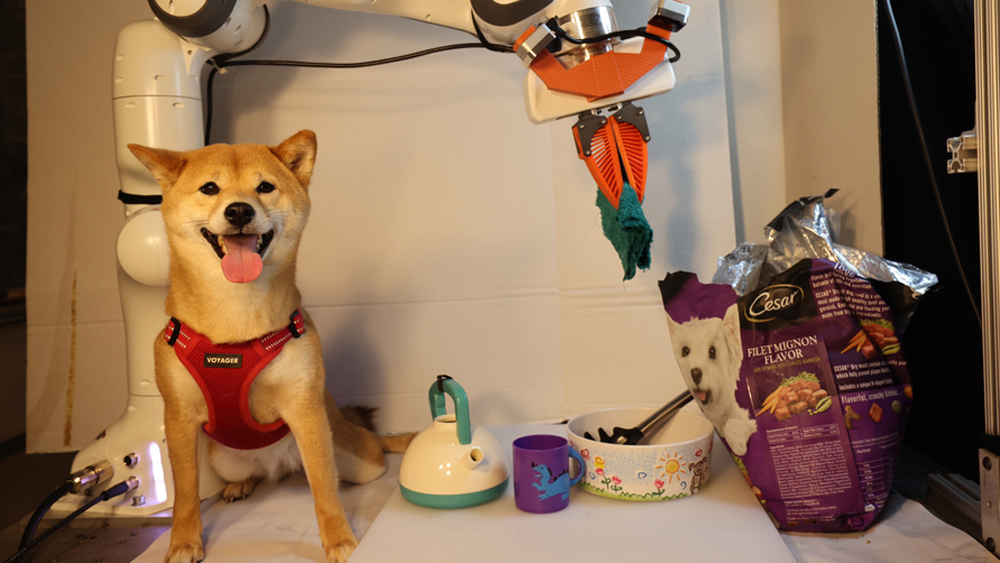
With enough of the right kind of data you can teach new robots new tricks. Photo by Possessed Photography on Unsplash
In the 1960s cartoon series The Jetsons, Rosie the robotic maid seamlessly switches from vacuuming the house to cooking dinner to taking out the trash. But in real life, training a general-purpose robot remains a major challenge.
ADVERTISEMENT |
Typically, engineers collect data that are specific to a certain robot and task, training the robot in a controlled environment. However, gathering these data is costly and time-consuming, and the robot will likely struggle to adapt to environments or tasks it hasn’t seen before.
To better train general-purpose robots, MIT researchers developed a versatile technique that combines a huge amount of heterogeneous data from many sources into one system that can teach any robot a wide range of tasks.
Their method involves aligning data from varied domains, like simulations and real robots, and multiple modalities, including vision sensors and robotic arm position encoders, into a shared “language” that a generative AI model can process.
By combining such an enormous amount of data, this approach can train a robot to perform a variety of tasks without having to train it from scratch each time.
…
Add new comment