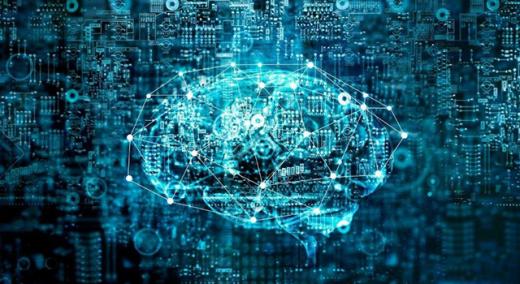
Throughout human history we’ve constantly sought out tools and capital to make us more productive. From the formation of basic tools to assist in farming to real cultivation and shaping of the land for greater yields, humankind learned to grow food. Further research into genetics, fertilizers, and pesticides enabled us to rapidly scale food production. From early sweatshops to almost fully automated factories, we’ve learned how to scale manufacturing and get far more productivity from fewer workers and more machinery and automation.
ADVERTISEMENT |
In this manner, we’ve learned to improve the deployment of human labor, land, tools, machinery, and other capital to improve our quality of life. Now, we must fully engage the asset that we have the most of that is producing the least for us: data. It’s time to put our data to work.
…
Add new comment