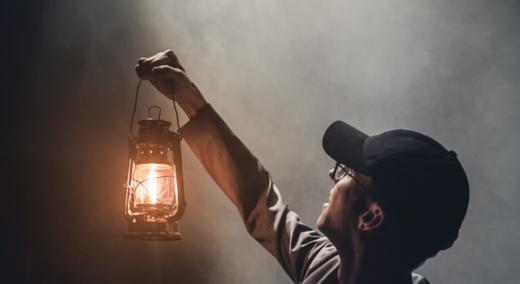
Since the start of the Covid pandemic I have received many questions about how to analyze the Covid numbers using process behavior charts. Various schemes have been proposed and used. This column will discuss appropriate ways of analyzing data from epidemics and pandemics.
ADVERTISEMENT |
Now to be clear, in this article the term “data analysis” is distinct from mathematical modeling. Epidemiological models incorporate subject-matter knowledge to create mathematical models that are useful for understanding and predicting the course of an epidemic. These models allow the experts to evaluate different treatment approaches. While these models are generally refined and updated using the collected data, this is not the same as what I call data analysis. Data analysis can be carried out by non-epidemiologists. This occurs when people try to use the data to tell the story of what is happening. This article is about the analysis of the existing data by non-epidemiologists. Nothing in what follows should be construed as a critique of epidemiological models.
…
Add new comment