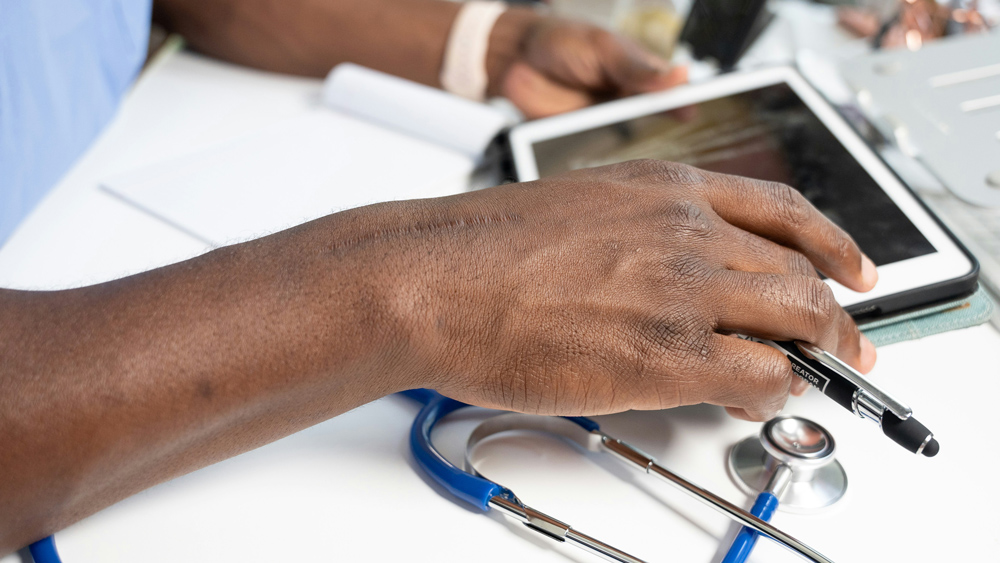
AI has been part of healthcare technology for a long time. Image by Nappy on Unsplash
Many people don’t realize just how long AI has been around in the healthcare industry—and are surprised to find out that it’s something that’s been relied on for 50 years already.
ADVERTISEMENT |
MYCIN, a computer-based model with machine learning capabilities, was developed by a team of researchers at Stanford University in the early 1970s. It marked a significant milestone in the intersection of artificial intelligence and healthcare. The project was led by Dr. Edward Shortliffe, who aimed to diagnose bacterial infections via MYCIN and recommend appropriate antibiotic treatments based on patient-specific data.
The system operated by asking physicians a series of questions about the patient’s symptoms and laboratory results, using a knowledge base derived from expert consultations.
…
Add new comment