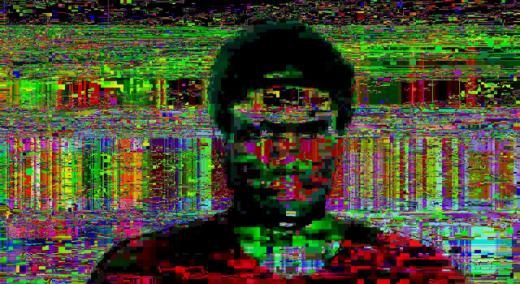
"Skin Cells" Credit: Antonio Roberts
In our constantly evolving, data-rich universe, collecting, interpreting, and understanding process data can be tricky. But it is increasingly important if we want to maintain sustainable quality across product development and manufacturing processes. This challenge is particularly evident in the life sciences arena, where pharmaceutical, biotechnology, and medical device manufacturers constantly strive to build quality processes that deliver “fit for purpose” output.
ADVERTISEMENT |
Process data typically come from a collection of diverse sources in varying formats. These data are dynamic, which often means they have a short shelf life. The longer a piece of data sits, the greater chance that it loses relevancy. Data often are also coming from an extremely complex supply chain that may include development or manufacturing partners’ systems and processes.
…
Add new comment