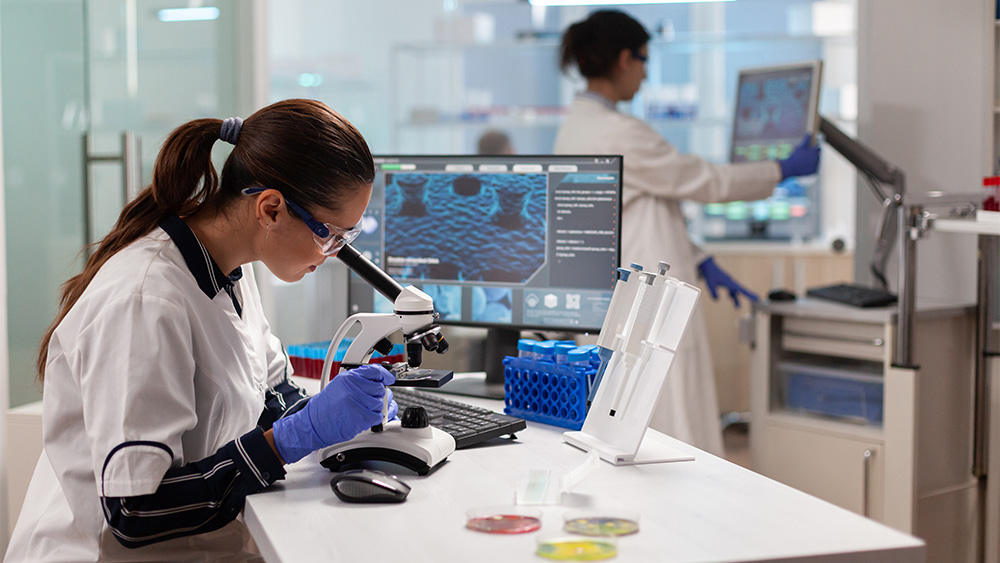
Before the ICH Harmonized Tripartite Guideline Q9—“Quality risk management”—was introduced in 2005, the pharmaceutical industry was evolving but lacked a structured, scientific, and systematic approach. Various stakeholders, including the industry, regulators, and patient rights groups, recognized the need for a methodical risk assessment process. However, clear guidance was missing.
ADVERTISEMENT |
Previous to the ICH Q9 framework, risk assessment approaches were characterized in three ways: 1) they were reactive, addressing issues only as they emerged rather than proactively identifying and mitigating risks; 2) they were variable, with significant differences in practices across companies and regions leading to inconsistencies in drug safety and quality standards; and 3) they had limited formalization. Although some risk management activities were present, there was no comprehensive, formalized approach that fully integrated risk management into all aspects of pharmaceutical development and manufacturing.
…
Add new comment