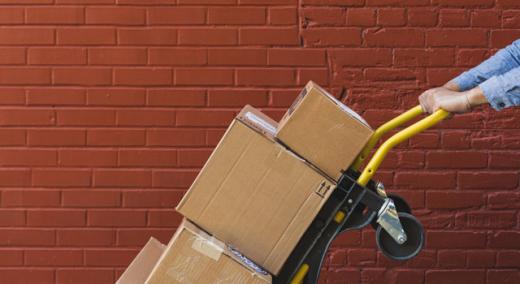
Body
In today’s highly competitive business environment, a trusted supply chain that functions seamlessly is essential. Even small errors can affect the entire supply chain, resulting in lost revenue, unsatisfied customers, and irreparable damage to your brand.
ADVERTISEMENT |
Now more than ever, companies need the ability to make informed decisions quickly and accurately to stay ahead of their competitors. Predictive analytics has emerged as a game-changer in this regard, allowing organizations to gain a competitive edge by making data-driven decisions.
…
Want to continue?
Log in or create a FREE account.
By logging in you agree to receive communication from Quality Digest.
Privacy Policy.
Add new comment